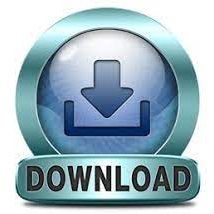

It is therefore desirable to come up with a more adequate relative description of approximation error. However, in a 1 frequent situation when the actual value is 0, the relative error does not make sense: no matter how close is our estimate xe to 0, the relative error is infinite. When the actual value is positive or negative, relative error makes perfect sense: the smaller the relative error, the close the estimate xe to the actual value x. The problem with the usual definition of a relative error
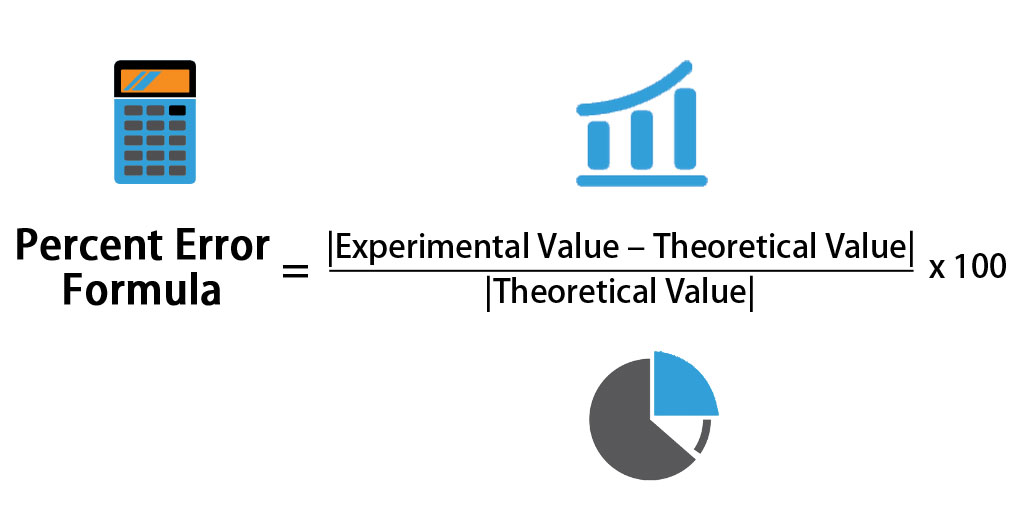
Such an upper bound serves as a description of approximation accuracy: we can say that we have an approximation with an accuracy of ☐.1, or with an accuracy of 5%.
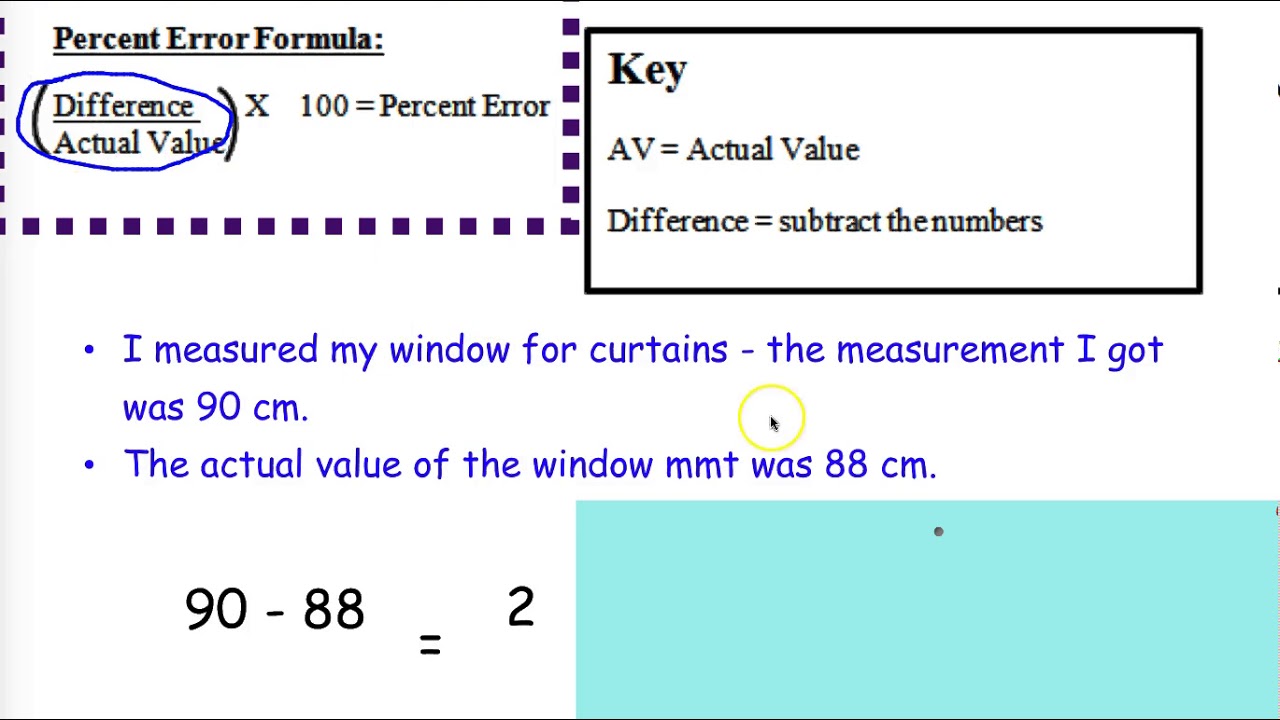
Often, however, we know the upper bounds on one of these errors (or on both of them). Since the actual value x is now known exactly, we cannot compute the exact values of the corresponding errors.
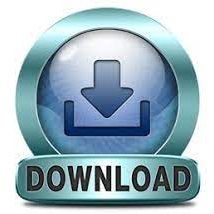